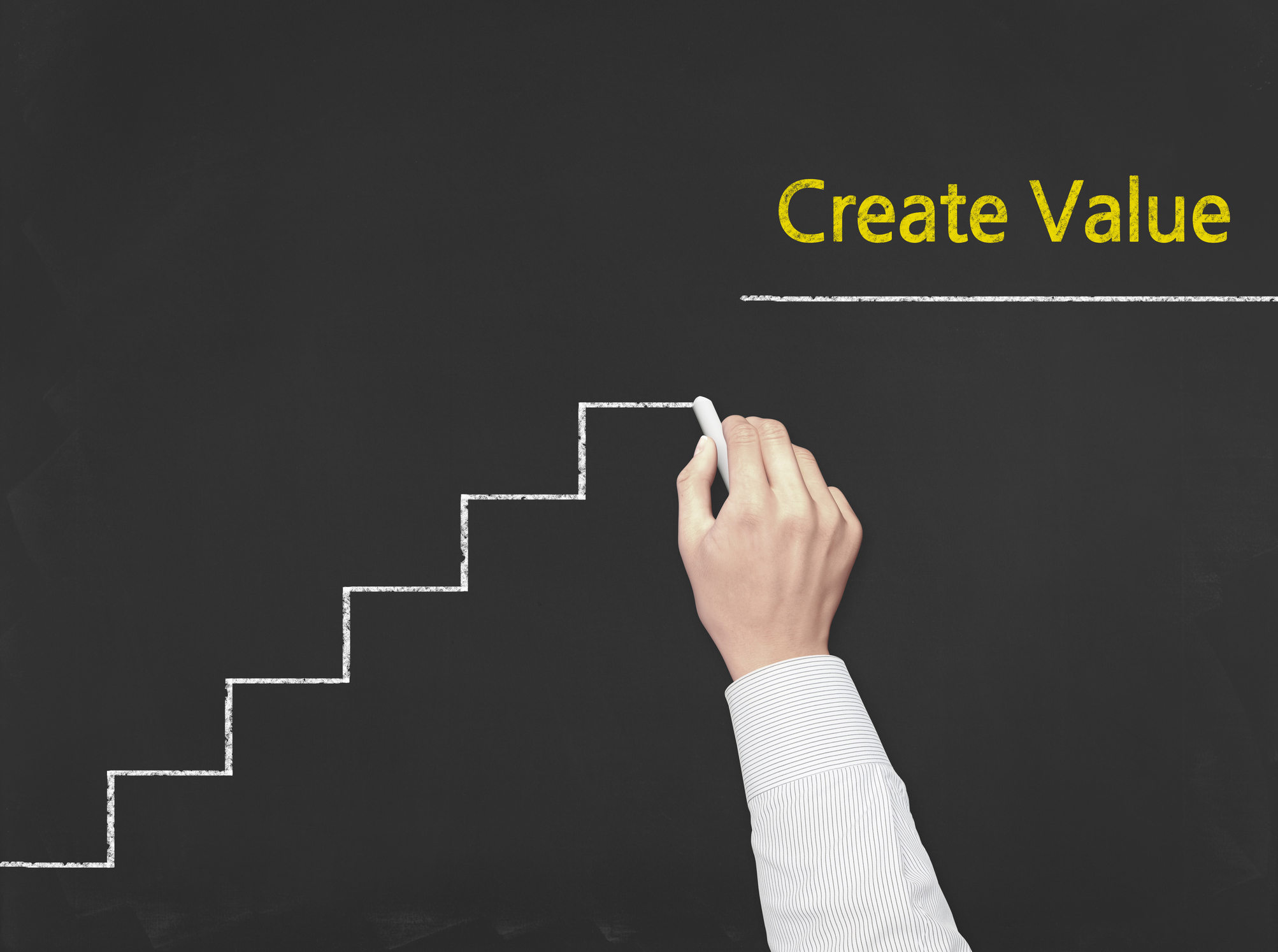
HOW TO MOVE FROM AI PROOF OF CONCEPT
TO AI DELIVERY AT SCALE
It’s time for the public sector to keep pace with AI investments and deliver meaningful service improvements
When I joined Pivotl I had only recently finished working on the first public facing LLM for the UK Foreign Office and rather naively – as it turns out – assumed that many other government departments would follow suit and begin embracing the benefits of AI beyond a Proof of Concept. However, as I began to research the state of AI practice across the sector, I quickly discovered that the project I was involved in delivering was unique, rather than being the norm.
In this blog I’ll talk about what needs to be done to move from a Proof of Concept into scaleable delivery of improved services for users, providing tangible guidance into how to get up and running. Moving beyond the hype and into practical application.
But firstly, here’s three AI success stories from both inside and outside of the public sector to demonstrate what others have already achieved.
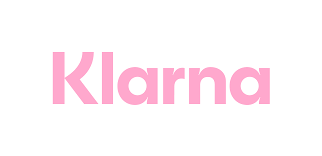
Augmenting customer services, marketing and internal knowledge with AI at KlaRna
If you’re interested in pragmatic uses of AI then I urge you to listen to how Klarna, the FinTech company embraced the use of AI. The Training Data podcast interviews Sebastian Siemiatkowski the CEO of Klarna, who shares in great detail about how Klarna embraced AI – something that he personally strongly encouraged across the company, setting teams up to succeed.
He tells stories of how the use of AI has:
- removed backlog for the customer service department,
- provided a self-serve questioning tool for internal employees, and
- helped augment the creativity of those in marketing.
Their ‘strategic advantage’ he claims, is now down to AI.
Although the public sector operates different from a commercial business, it’s important not to dismiss Klana’s AI experience; after all, one of the Government’s strategic objectives is to deliver high quality services for users, and AI can have a huge role to play in achieving it.
Financial benefits of GAI at Walmart
AI is having significant business results. Walmart stock rose to record earnings, with the CEO Doug McMillon attributing some of that to the fact that Walmart was finding “tangible ways” to leverage generative artificial intelligence to improve customer, member and employee experiences.
We are seeing this more and more, and even if you’re an AI sceptic, it’s difficult to deny that it’s something large organisations need to develop. This means going beyond a trial or a proof of concept or re-inventing the wheel, but actually using AI in current, existing services to improve the quality outcomes for users today.
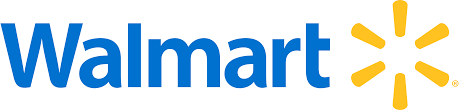
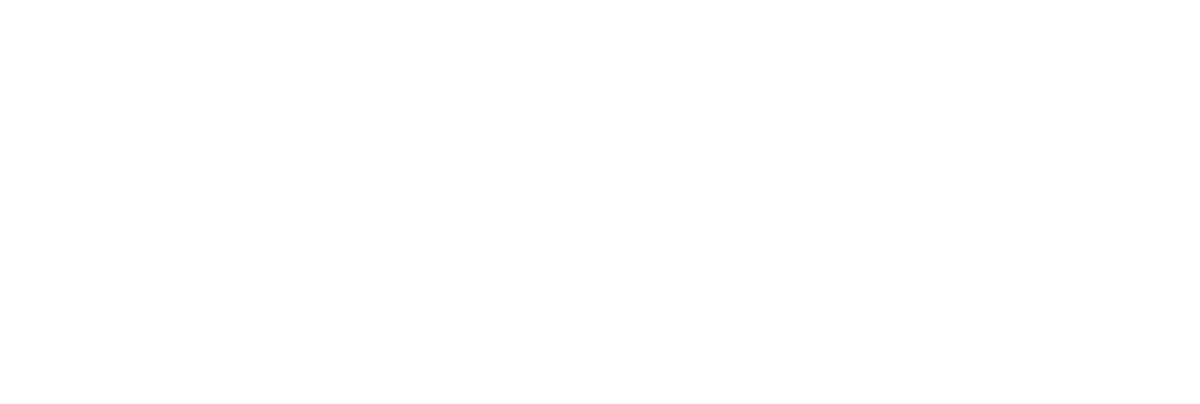
Driving innovation at the FCDO
The UK Foreign, Commonwealth & Development launched a public facing LLM several months ago in their customer services team – the benefits of which are believed to have had significant improvements in customer experience and efficiency.
Keeping pace with service users expectations
As more and more businesses adopt AI and consumers become accustomed to the augmented experiences, the base line expectations of public service users will over time increase. It won’t simply be enough to provide digital services as they are today. It’s great to see the FCDO take up the mantle and launch the UK’s first public facing LLM in government.
1. Be pragmatic – focus on iteration not transformation
The most sensible use of AI tools right now is to augment delivery of government services. Start with identifying a problem that is easy to define and you’re clear on what you want to achieve. Are your people spending too long reading, writing, seeing, learning… AI can augment services by doing these things faster, simpler and cheaper. That’s AI. That way you can remove the hype by thinking in these simple terms in a service context.
When I worked on the Foreign Office AI project, we focused on how to improve the contact-based service. AI was simply the tool we used to alleviate some of the friction between users and agents.
This is service iteration, not service transformation.
2. Get buy in from all levels of the organisation
Run “art of the possible” sessions with a mix of leadership and front-line people, to identify your most pressing issues or potential AI use cases and evaluate the possibilities. This approach aids learning, engagement and buy in.
We run these with customers quite often, across data, cloud and AI. It’s about blending the expertise in the room with experts who know what AI is capable of and bringing these together, then prioritising. Put simply, AI is a set of tools, they are just different and smarter to the tools we had before.
3. Invest in data for reliable, robust and scalable AI
When we recently delivered a hugely successful AI project in healthcare, we started with a data platform bringing in 100+ data sources to create a really clear picture of how we could help users. It doesn’t always need to use hundreds of data sources, but it’s important to have confidence in your data given its reliance on developing powerful and reliable AI tools.
An analogy that I stole from this excellent talk by Cassie Kozyrkov is that Data is the learning material and AI is the student – the more accurate the data, the better the student will perform. And the beauty of a data platform is that it can be configured to ingest multiple data sources which means it can scale with your future needs.
The time is now for the public sector to demonstrably use AI in services, to keep pace with the private sector and the expectations of users. We can deliver incredible service augmentation right now across the sector using a combination of internal and external skills and know-how together in a coherent way.