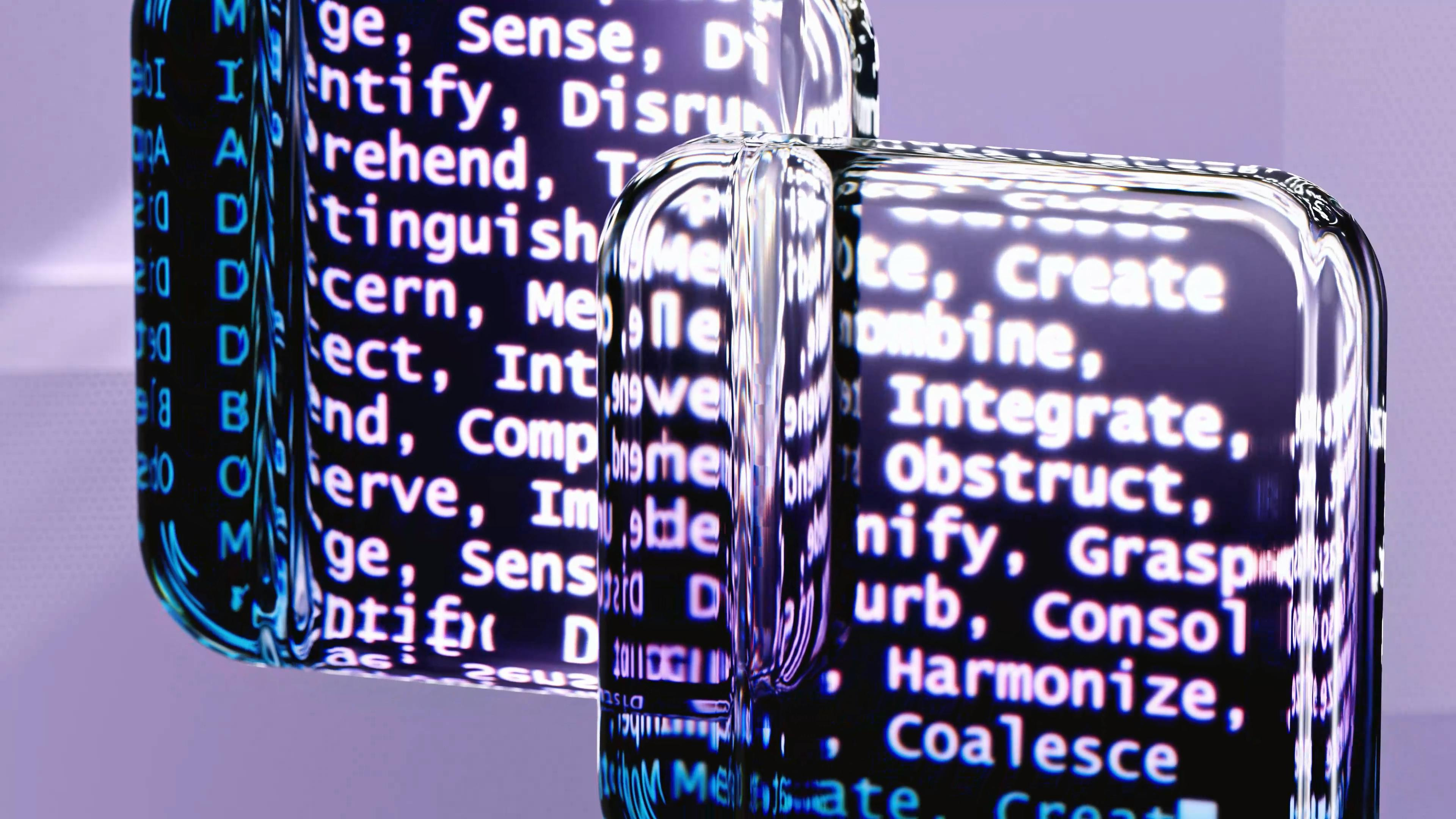
Time to embrace semantics – getting your data ready for AI
The great executive leadership cries of the mid 2020’s – “Why aren’t we scaling AI?” “Why are we stuck at Pilots?”. “Microsoft tell me it’s easy – why are we making such heavy weather of it?”.
The answer is partly semantic. Not in the usual sense of the word but in the sense that a high performing organisation with data at its heart would be using a “Semantic Layer” to accelerate and unlock AI’s potential.
This blog post isn’t going to explain semantic layers – there are some great online resources that can do that better than me. Like this one from DataCamp:
What is a Semantic Layer? A Detailed Guide | DataCamp
Rather, this is a post about the systemic and cultural challenges of semantic layer development in complex, public service organisations such as Councils or Health authorities. This isn’t an anti-semantic layer argument, but it is a call to action to that same, frustrated, leadership cadre who want their organisations to exploit the full potential of data and AI. They are essential to semantic success or semantic frustration.
The great executive leadership cries of the mid 2020’s – “Why aren’t we scaling AI?” “Why are we stuck at Pilots?”. “Microsoft tell me it’s easy – why are we making such heavy weather of it?”.
Semantic Challenges in Public Service Delivery
Public sector organisations generate huge amounts of data that should, in theory, be analysed in a consistent way to make essential decisions – either by humans or by computers. The data come from multiple sources and in different formats making it hard for non-data professionals – users – to determine its meaning and importance. In public service the data often supports decisions about vulnerable people, the importance of correct interpretation can’t be overstated.
Those of us who have worked in complex public service organisations know they operate with fluid naming conventions and definitions in just about everything they do. Taxonomies are in a state of constant evolution with new verbs and nouns being added to groups of classified terms. Furthermore, the relationships between terms changes over time and between different professionals. Professionals apply ‘professional judgement’ to what things are called and how they interact with each other in a fluid way. The result is an environment where semantic debate is rife in most interactions. Seeking clarification takes energy and effort. This diverse vocabulary becomes hard coded into structured and unstructured data.
Combine this fluidity with minimal data standardisation (which is usually common, sometimes non-existent in many of these organisations) and the result is significantly limited automation and assurance in current data transformation activity. Working on behalf of complex professional led services, analysts are forced to compensate by overwriting the code in their data models with alarming frequency as semantic definitions aren’t locked down. A level of manual resource demand and poor-quality control, which cannot be scaled to work with AI.
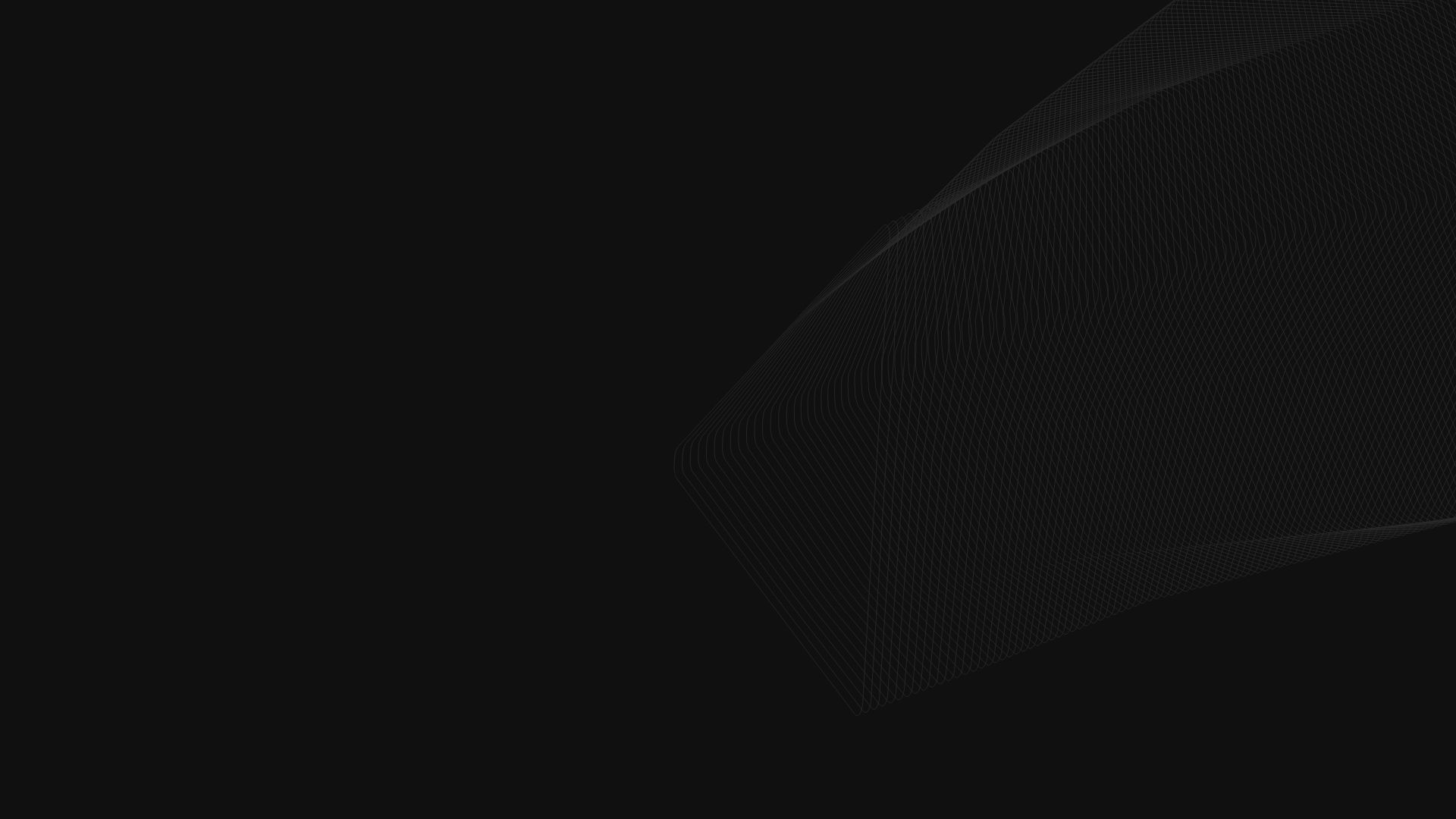
The Semantic Opportunity
This is where the semantic layer comes in. The semantic layer sits between where data is stored, and the IT applications used by end users. It defines the rules and relationships between the data elements and provides a common vocabulary for the data – in clear, business terms. The public sector professional can then easily interact with the data, without needing technical knowledge of the data sources. If we get our semantics right, we can automate data processing, build better, impactful outputs and work with greater assurance of appropriate and legitimate data use.
The Importance of a Strategic Data Platform
Data and AI platforms, aligned to the right Operating Model of accountable, capable people working to agreed policies, processes and protocols are crucial here. The right platform provides a preconfigured environment in which semantic descriptors and associated definitions can be recorded and built into relational processing routines. These can support automation across all types of data processing to underpin workflows, all types of analytics and everything in the AI multiverse.
But there’s no such thing as a free lunch, right? To successfully deploy this layer, leadership and other professionals must support tighter taxonomies of plain English terms across the entities, activities, inputs, and outputs they work with. This includes being clear on how these are defined, how they relate to each other and making sure this is documented and remains as consistent as possible between individuals and teams. This is as much the responsibility of service teams as any data management or AI development functions. Building time and resource into this semantic definition rigour is critical – time can only be unlocked by leadership via their strategic prioritisation.
It is true that AI itself can help with this. Tools like cognitive search agents bring greater capacity and capability to addressing the lack of agreed and fixed semantics in legacy data sets. So, before we use semantic layers to support AI, we can use AI to help build our semantic layers. However, this is a significant investment, and one that would be questionable without a shift in culture, required to not keep exacerbating the problem in service practice – again another leadership task.
If you are an exec who wants to scale AI….
Invest in a platform, embrace semantics, get on top of definitions, – this, much more than your personal knowledge of Deep Seek or Chat GPT, will unlock massive value for your users, staff, and the taxpayer.